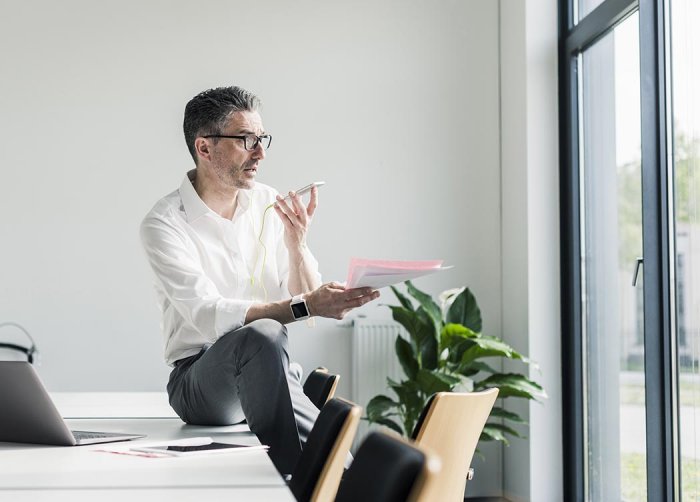
How does Sofrecom accompany you?
Telecom operators are part of one of the most solicited industries on customer relations channels. Conversational agents or 'chatbots' are proving to be an ideal ally to contain incoming calls and improve customer satisfaction. As a proof, Orange has announced a target of 50% of customer interactions managed by its chatbot Djingo in 2023, while Vodafone has set a target of 60% by 2021. By becoming the essential gateway to human support, chatbots create a form of collaboration with customer advisors, which will evolve as conversational AI progresses.
Chatbots, an indispensable and critical asset in customer relations
The emergence of smart robots capable of interacting in natural language with clients has paved the way for a new standard in the digital experience offered by the operator. By simplifying access to digital paths and ensuring 24/7 availability, virtual agents have become an indispensable lever of customer autonomy for account management, assistance or complaints. In France, Total Direct Energie estimates that chatbots have contributed to 40%11 of calls avoided.
The specificity of the conversational experience is to create a very strong polarization of customer satisfaction. Chatbots are a layer of intermediation with human support. They therefore represent a critical component of the customer experience, requiring significant investment to make them effective in understanding customer intentions, adapting them to the experiential codes of the channels on which they are deployed, and integrating them with the company's information systems to offer a personalized customer experience.
The new sources of value generated by voice and vision
If chatbots first appeared in the form of a text conversation window (chatbot), voice chatbots (VoiceBots or Callbots) may generate even more value.
Indeed, 40% of customer service calls could be automated thanks to AI12 while customer service calls still represent about 55% of the flows13 and they have not decreased drastically in the last years in France.
The first challenge is therefore to improve customer autonomy on interactive voice interfaces (IVR) by allowing customers to express themselves naturally and by offering personalized voice paths thanks to CallBots. With 50% of customers dissatisfied with their IVR experience and only 14% of IVRs embedding natural language understanding14, the opportunity is huge. And the technologies are mature: Isabelle, Bouygues Telecom's CallBot in France, for example, supports 150 customer intentions and the customer autonomy rate reaches between 60% and 90% on simple calls according to the provider Calldesk15. The second challenge is to make it acceptable to customers to be called by CallBots. A use case is deployed by Orange in Eastern Europe with the Lekta AI solution for bill collection, in countries where it is common for a customer advisor to call for this type of action.
But for the main reasons of contact in customer service of an operator, i.e. installation help, technical assistance on internet boxes and bill explanation, the text or voice experience can be limited. For these customer cases, the implementation of vision on bots, thanks to the artificial intelligence technology 'Computer Vision', becomes crucial in a context of boom of video chat in customer service. By coupling computer vision to augmented reality, the user experience for installation or troubleshooting on internet boxes is simplified thanks to the indications on the connections to be made in superposition of the equipment filmed by the smartphone.
Augmented customer advisor
The other side of customer relationship virtualization is the impact on the customer advisor's job. The chatbots currently deployed by operators are capable of handling more than half of the conversations autonomously. The customer advisor's job is thus focusing on complex technical issues and augmenting it with new capabilities to achieve this.
This form of chatbot-customer advisor collaboration can be found, for example, in the ability of conversational AI to detect a customer's negative feeling and refer him to an advisor (e.g. Orange Bank with IBM Watson technology), to route customer requests to the most qualified agents (affinity matching), to issue recommendations or to transcribe conversations in real time. The advisor can even invite a chatbot into his or her conversation with a customer, as proposed by certain customer relations solutions such as LivePerson.
The result will be a shorter processing time and first contact resolution as well as a greater relevance of sales proposals thanks to a better focus of consultants on their technical and commercial skills rather than on tasks related to back-office processes.
Towards a bi-directional collaboration between customer advisors and chatbots?
This collaboration remains unidirectional for the time being; customer advisors cannot enrich the knowledge of a chatbot without risking altering the performance of the conversational AI. Training a chatbot remains an expert activity and advisors can at best collaborate with development teams.
This AI, based on a machine learning architecture called "Transformer", is capable of creating answers from scratch by relying on knowledge acquired in other contexts (transfer learning). This means that it will be able to manage increasingly complex customer contact patterns autonomously, without necessarily having been pre-trained on these patterns.
Unlike the AI technologies commonly used in Natural Language Processing, generative conversational AI feeds on unstructured data and can therefore be trained with huge databases with limited effort.
Amazon, for example, tested generative conversational AI in the context of service in 2020 after testing it with 5 million responses from 350K customer chats, which is the equivalent of several years of experience for a customer advisor16.
In addition to having demonstrated a contribution in the flexibility of the training process and an improvement in customer autonomy, this experimentation materialized a probable evolution of chatbot-customer advisor interactions towards a bi-directional collaboration. Indeed, during this test, the advisor could select or modify an answer proposed by the AI without putting at risk the global understanding of the chatbot. In addition to saving time for customer advisors, this shows that customer advisors could increasingly support chatbots in their customer interactions. Going further, we could imagine chatbots personalized by each customer advisor according to their own editorial and behavioral style (on the model of the Replika.ai service) and thus becoming true digital doubles.
Today, chatbots and AI are deployed in various forms across all customer relationship channels to handle simple customer cases and support customer advisors in handling complex cases. New generative conversational AI technologies could change this paradigm by enabling bots to handle increasingly complex cases and by giving customer advisors an active role in improving the quality of the bots' interaction. We could see the emergence of fully hybrid conversational agents where each component - human or digital - would augment the other. For the time being, operators must accompany these advances in conversational AI, which are synonymous with productivity gains and customer satisfaction. This requires to engage now in a transformation of the Customer Relationship orchestrated by AI.
Extract form ou white paper : Challenges and advancements in the era of data and artificial intelligence
1 Do you Dream Up, 2020
2 Nice Incontact, 2020
3 BVA - Observatoire des Services Clients 2020
4 Nuance, 2020
5 Calldesk 2020
6 Amazon Blog science, 2020
Extract from our white paper : Challenges and advancements in the era of data and artificial intelligence