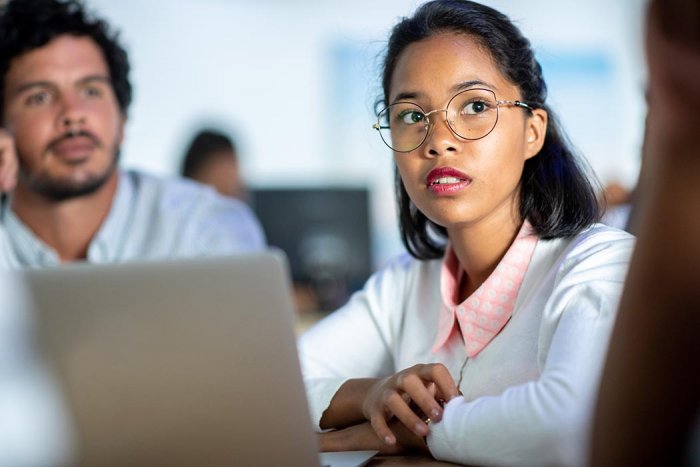
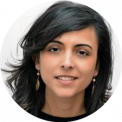
While the current crisis provides an opportunity to demonstrate their value, it also highlights the problems of their governance.
Stefania Rubrichi, Erwan Le Quentrec and Zbignew Smoreda
The COVID-19 pandemic has presented to the world unprecedented challenges, calling on countries to take urgent and decisive action to address the health emergency and mitigate the associated economic and social consequences. In this context, mobile data has become a crucial source of support for decision-making.
Governments, public health authorities, and scientists are strongly committed to rapidly developing solutions to better address and combat coronavirus infections. These actions include the development of diagnostics, treatments and vaccines, as well as the use of technology and digital data. Among these, mobile phone data is part of the crisis management system. Federated by the Challenge Data for Development24 and the flagship conference NetMob25, the data generated by our cell phone uses have become one of the leading sources of accurate, near-real-time data for studies of human presence, mobility, and social interactions26. In particular, their utility has been proven in many epidemiological settings27 28 29 30 31 32.
Within days of the start of the epidemic, during the first wave, there was considerable pressure from a broad community of scientists who mobilized and called on governments and telecommunications companies to act. They argued for the need to access aggregated human mobility data33, and cell phone data in particular34, as a crucial source of information to better understand and control the spread of the COVID-19 virus.
At the same time, in March 2020, the European Commission promoted initiatives to mobilize anonymized and aggregated telecommunications data from EU mobile operators in the fight against COVID-1935. Subsequently, in April, it published a roadmap for the gradual lifting of containment measures and supported the collection and use of this data to manage it36.
As the epidemic worsens and containment policies are implemented, researchers and private companies around the world have joined forces and started working together to manage the epidemic. Some have focused on detecting and locating close contacts of infected people, others on identifying transmission 'clusters' or evaluating mobility restrictions and social interactions 37 38 39 40 41 42 43 44.
Orange Group, too, immediately deployed a taskforce to contribute to the COVID19 cause with several actions around the use of telecommunication technologies.
In this article, we report on a data sharing and use initiative between private and public sector actors in France that brought together researchers from the French National Institute of Health, Medical Research (INSERM) and Orange Labs in a collaborative research project. We review the main results obtained so far and analyze the issues, limitations and implications of this type of initiative.
The EVALCOVID-19 collaborative project
In March 2020, when the French National Research Agency allocated exceptional funding for a call for research on the epidemic45, the INSERM-Orange Labs consortium was able to respond quickly with the EVALCOVID-1946 project proposal, building on a collaboration already initiated on the Ebola epidemic in Africa.
This project proposes to use aggregated indicators on population mobility built in near-real time from the cell phone network to inform public health authorities throughout the successive phases of the epidemic.
The work began in the second half of March when the epidemic took hold in France with exponential dynamics. During this acceleration phase, heavy restrictions on mobility and social contacts through national containment were implemented, to delay and contain the epidemic and relieve the pressure on the health system. At this stage, mobility analysis is essential to identify the behavioral and societal dynamics that shape but also limit the extent of the restrictions. To do so, we used indicators provided by Flux Vision47 (Orange Business Services) and studied changes in French people's mobility behaviors in response to the epidemiological situation and the interventions imposed by the French government, at different spatial and temporal scales48.
This study showed that confinement strongly reduced work- or school-related mobility, especially during peak hours, as well as long commutes. But these decreases were variable across regions because of demographic and socioeconomic factors as well as differences in industries (fig. 1).
Figure 1. Mobility reduction induced by the first containment: (a) Mobility reduction by age, time of day, and (b) region; (c) mobility reduction by demographic, socioeconomic, and epidemic indicators.
In addition, significant reductions were observed in regions with the highest level of epidemics, suggesting that awareness of the risks may have strengthened the follow-up of recommendations. Highlighting these explanatory factors is important in order to best define current and future movement restrictions.
During the decelerating phase of the epidemic, when the peak of infections was reached, the results of this first study were used immediately to precisely parameterize a stochastic transmission model based on age profile and social contact data49. This model made it possible to (i) assess the situation of the COVID-19 pandemic in France according to short-term projections; (ii) assess the impact of containment on viral dissemination; (iii) explore possible exit strategies, in terms of type of intervention (which ones, which combinations), time (best time to implement them, duration) and space (which regions, departments); (iv) assess the capacity needs of the health system for all the epidemic situations below (fig 2).
This research, published quickly, was made available to public health authorities. When lockdown was eased in spring 2020, this work did not stop in order to monitor and anticipate possible rebounds.
As of the moment the article was written (early 2021), a new wave of the epidemic has hit the country, forcing authorities to introduce further restrictions. On October 17 and 24, a curfew was gradually implemented in 54 departments of mainland France. A second generalized curfew was implemented from October 30 to December 13, 2020. A national curfew is currently in place. Further restrictions are likely in the future, as vaccination is just beginning and new variants are circulating50. The measures taken and their acceptance may change rapidly, justifying the perpetuation of the tools built 51.
Figure 2. Analysis of the impact of the first confinement on epidemic activity in Île-de-France: (a) Calibration of the model on daily hospitalization data in Île-de-France before confinement and projections for the phase containment; (b) projections of the number of ICU beds occupied during confinement.
Access and sharing of personal data: open ended questions
In this context, it is clear that rapidly delivered mobility indicators are an essential source of information for policy makers and authorities. While the current crisis provides an opportunity to demonstrate their value, it also highlights the problems of their governance. These indicators, built from our clients' uses, are very sensitive in terms of their collection, storage, use and even sharing. All of this raises legitimate questions in legal, economic and ethical terms52.
In the context of this collaborative research, only the aggregated, anonymized mobility indicators - in the form of origin-destination matrices - needed for the explicitly defined objectives of the project are shared between the two institutions. More precisely, these indicators are produced by the commercial solution Flux Vision, based on the on-the-fly processing of cell phone data previously anonymized in accordance with strict privacy requirements, audited by the French data protection authority (CNIL, Commission Nationale de l'Informatique et des Libertés).
This pre-existing solution provided an immediate response to the crisis. However, it has some limitations.
It was initially designed taking into account regulatory and technological requirements, particularly the e-Privacy Directive53 and its national transposition.
Moreover, as a proprietary solution, the entire process of their construction is not immediately shared, which may raise questions 54 55 56. As the authors of the report Using Data to fight COVID-1957 illustrate: "Some telecom companies, such as Telefonica with SmartSteps, Orange with FluxVision or Vodafone with Vodafone Analytics, have developed commercial systems and products. However, these solutions are proprietary and closed, so it is difficult to base public policies on them. At the same time, the idea of transparency also comes into tension with intellectual property law, as algorithms are akin to a trade secret, the disclosure of which could jeopardize a business model 58.
Finally, as with any system designed to meet other needs (in this case the tourism or transport sectors), the indicators are not necessarily always adapted to the specific context of the current epidemiological crisis. Adjustments must therefore be tested.
This pre-existing solution allowed an immediate response to the crisis. It was initially designed with regulatory and technological requirements in mind, particularly the e-privacy directive and its national transposition. Moreover, as a proprietary solution, the entire process of their construction is not shared from the outset, which may raise questions. As the authors of the report Using Data to fight COVID-19 illustrate: "Some telecom companies, such as Telefonica with SmartSteps, Orange with FluxVision or Vodafone with Vodafone Analytics, have developed commercial systems and products. However, these solutions are proprietary and closed, so it is difficult to base public policies on them. ". At the same time, the idea of transparency also comes into tension with intellectual property law, as algorithms are akin to a trade secret whose disclosure could jeopardize a business model.
Finally, like any system designed to meet other needs (in this case the tourism or transport sectors), the indicators are not necessarily always adapted to the specific context of the current epidemiological crisis. Adjustments must then be tested.
A complementary approach could be based on secure access to pseudonymized data - in this case the call reports (CRA) already collected for billing reasons - following a 'privacy-conscious' usage model proposed by some researchers59. For example, it could be useful to mobilize indicators that aim to better understand the problem of mixing populations in time and space or to better qualify the semantics of displacement. Indicators that would be constructed in a transparent manner with all stakeholders on the one hand, while respecting the confidentiality of clients thanks to advances in algorithms on the other60. Despite numerous initiatives in recent years on best practices for a new model of sharing and using pseudonymized data that respects privacy, regulatory obstacles remain.
The current health crisis has reminded us of the urgency of addressing this issue61. On February 10, 2021, the Council of the European Union, which brings together the ministers of the member states, reached a consensus for a final text on e-privacy regulations which authorize the use of metadata, in particular to monitor epidemics and their spread or in humanitarian emergencies62. It must, henceforth, be submitted to the European Parliament for deliberation and vote.
This ongoing research project is a reminder that the data in our networks contains singular uses that are also part of our commitment as a socially responsible company. Just like many projects were initiated out of a sudden as a result of an unknown disease, this one has condensed different issues and controversies around the use and sharing of personal data for research purposes.
Extract from our white paper : Challenges and advancements in the era of data and artificial intelligence
1 “NetMob 2019, 8-10 July Mathematical Institute, Oxford University, Oxford, UK.” https://netmob.org/#pasteditions (accessed Jan. 28, 2021).
2 V. Blondel, A. Decuyper, and G. Krings, “A survey of results on mobile phone datasets analysis,” EPJ Data Science, vol. 4, Feb. 2015, doi: 10.1140/epjds/s13688-015-0046-0.
3 L. Bengtsson et al., “Using mobile phone data to predict the spatial spread of cholera,” Sci Rep, vol. 5, p. 8923, Mar. 2015, doi: 10.1038/srep08923.
4 F. Finger et al., “Mobile phone data highlights the role of mass gatherings in the spreading of cholera outbreaks,” PNAS, vol. 113, no. 23, pp. 6421–6426, Jun. 2016, doi: 10.1073/pnas.1522305113.
5 M. Tizzoni et al., “On the Use of Human Mobility Proxies for Modeling Epidemics,” PLOS Computational Biology, vol. 10, no. 7, p. e1003716, Jul. 2014, doi:10.1371/journal.pcbi.1003716.
6 A. Wesolowski et al., “Quantifying the Impact of Human Mobility on Malaria,” Science, vol.338, no. 6104, p. 267, Oct. 2012, doi: 10.1126/science.1223467.
7 A. Wesolowski et al., “Impact of human mobility on the emergence of dengue epidemics in Pakistan,” Proc Natl Acad Sci U S A, vol. 112, no. 38, pp. 11887–11892, Sep. 2015, doi: 10.1073/pnas.1504964112.
8 S. Rubrichi, Z. Smoreda, and M. Musolesi, “A comparison of spatial-based targeted disease mitigation strategies using mobile phone data,” EPJ Data Sci., vol. 7, no. 1, Art. no. 1, Dec. 2018, doi:10.1140/epjds/s13688-018-0145-9.
9 C. O. Buckee et al., “Aggregated mobility data could help fight COVID-19,” Science, vol. 368, no. 6487, p.145, Apr. 2020, doi: 10.1126/science.abb8021.
10 N. Oliver et al., “Mobile phone data for informing public health actions across the COVID-19 pandemic life cycle,” Science Advances, vol. 6, no. 23, p.eabc0764, Jun. 2020, doi: 10.1126/sciadv.abc0764.
11 “European Commission tells carriers to hand over mobile data in coronavirus fight,” POLITICO. https://www.politico.com/news/2020/03/24/europe-mobiledata-coronavirus-146074 (accessed Jan. 28, 2021).
12 “Joint European Roadmap towards lifting COVID-19 containment measures,” Apr. 15, 2020. http://www.clustercollaboration.eu/node/16975 (accessed Jan.28, 2021).
13 “Valencia prepara un proyecto pionero con datos de móviles para trazar el movimiento del coronavirus | Tecnología | EL PAÍS.” https://elpais.com/tecnologia/2020-03-19/valencia-prepara-unproyecto-pionero-con-datos-de-moviles-para-trazar-elmovimiento-del-coronavirus.html (accessed Jan. 28, 2021).
14 B. Klein et al., “Assessing changes in commuting and individual mobility in major metropolitan areas in the United States during the COVID-19 outbreak,” p. 29.
15 M. U. G. Kraemer et al., “The effect of human mobility and control measures on the COVID-19 epidemic in China,” Science, vol. 368, no. 6490, pp.493–497, May 2020, doi: 10.1126/science.abb4218.
16 S. Lai et al., “Effect of non-pharmaceutical interventions to contain COVID-19 in China,” Nature, vol. 585, no. 7825, Art. no. 7825, Sep. 2020, doi:10.1038/s41586-020-2293-x.
17 “COVID-19 outbreak response: a first assessment of mobility changes in Italy following national lockdown | medRxiv.” https://www.medrxiv.org/content/10.1101/2020.03.22.20039933v2 (accessed Jan. 28, 2021).
18 M. Qian et al., “Oxford COVID-19 Imapct monitor,” Our World in Data. https://oxford-covid-19.com/ (accessed Jan. 28, 2021).
19 “Covid-19 UK Mobility Project,” Covid-19 UK Mobility Project. https://covid19-uk-mobility.github.io/Firstreport.html (accessed Apr. 17, 2020).
20 D. Martín-Calvo, A. Aleta, A. Pentland, Y. Moreno, and E. Moro, “Effectiveness of social distancing strategies for protecting a community from a pandemic with a data-driven contact network based on census and realworld
mobility data,” Complex Dig, 2020, Accessed: Jan. 28, 2021. [Online]. Available: http://ide.mit.edu/publications/effectiveness-social-distancing-strategiesprotecting-community-pandemic.
21 “Appel à projets Flash COVID-19,” Agence nationale de la recherche. https://anr.fr/fr/detail/call/appel-aprojets-flash-covid-19/ (accessed Jan. 29, 2021).
22 Ce projet s’inscrit dans le domaine de recherche d’Orange Digital Society sous la direction de Roxane Adle Aiguier (programme Transforming Society and Digital Humanities piloté par Christian Warocquier).
23 Les deux principaux piliers de la législation sur la protection des données personnelles dans l'Union Européenne sont le Règlement général sur la protection des données (RGDP), et la directive « Vie privée et communications électroniques » (e-privacy). Contrairement au RGPD qui est fondée sur la notion de gestion de risques, offrant ainsi des marges de manoeuvre pour définir des solutions sur mesure et qui permet aussi des exceptions explicites pour le traitement des données personnelles, tels que l'intérêt public, la recherche scientifique ou les statistiques publiques, la directive e-privacy limite explicitement ces conditions, en interdisant aux opérateurs d'utiliser leurs données sans le consentement des clients.
24 “Appel à projets Flash COVID-19,” Agence nationale de la recherche. https://anr.fr/fr/detail/call/appel-aprojets-
flash-covid-19/ (accessed Jan. 29, 2021).
25 “Flux Vision,” Orange Business Services. https://www.orange-business.com/fr/produits/flux-vision (accessed Feb. 05, 2021).
26 G. Pullano, E. Valdano, N. Scarpa, S. Rubrichi, and V. Colizza, “Evaluating the effect of demographic factors, socioeconomic factors, and risk aversion on mobility during the COVID-19 epidemic in France under lockdown: a population-based study,” Lancet Digit Health, vol. 2, no. 12, pp. e638–e649, Dec. 2020, doi:10.1016/S2589-7500(20)30243-0.
27 L. Di Domenico, G. Pullano, C. E. Sabbatini, P.-Y. Boëlle, and V. Colizza, “Impact of lockdown on COVID-19 epidemic in Île-de-France and possible exit strategies,” BMC Medicine, vol. 18, no. 1, p. 240, Jul. 2020, doi: 10.1186/s12916-020-01698-4.
28 GOV.UK, “Investigation of novel SARS-CoV-2 variant: Variant of Concern 202012/01,” GOV.UK. https://www.gov.uk/government/publications/investigation-ofnovel-sars-cov-2-variant-variant-of-concern-20201201
(accessed Jan. 29, 2021).
29 EPIcx lab INSERM, “COVID-2019 pandemic assessment,” EPIcx lab. https://www.epicx-lab.com/ covid-19.html (accessed Jan. 29, 2021).
30 E. Letouzé, N. Oliver, M. A. Bravo, and N. Shoup, “Using Data to Fight COVID-19 – And Build Back Better,” Nov. 2020. Accessed: Jan. 29, 2021. [Online]. Available: https://datapopalliance.org/publications/policy-paper-using-data-to-fight-covid-19-and-buildback-better/.
31 “Directive on Privacy and Electronic communications.” Accessed: Jan. 29, 2021. [Online]. Available: https://eur-lex.europa.eu/eli/dir/2002/58/oj.
32 J. Morley, J. Cowls, M. Taddeo, and L. Floridi, “Ethical guidelines for COVID-19 tracing apps,” Nature, vol. 582, no. 7810, Art. no. 7810, Jun. 2020, doi:10.1038/d41586-020-01578-0.
33 M. Taddeo, “The Ethical Governance of the Digital During and After the COVID-19 Pandemic,” Minds & Machines, vol. 30, no. 2, pp. 171–176, Jun. 2020, doi:10.1007/s11023-020-09528-5.
34 L. Floridi and M. Taddeo, “What is data ethics?,” Philosophical Transactions of the Royal Society A: Mathematical, Physical and Engineering Sciences, vol. 374, no. 2083, p. 20160360, Dec. 2016, doi:10.1098/rsta.2016.0360.
35 “cnil_rapport_garder_la_main_web.pdf.” Accessed:Mar. 17, 2021. [Online]. Available: https://www.cnil.fr/sites/default/files/atoms/files/cnil_rapport_garder_la_main_web.pdf.
36 Y.-A. de Montjoye et al., “On the privacyconscientious use of mobile phone data,” Scientific Data, vol. 5, no. 1, Art. no. 1, Dec. 2018, doi: 10.1038/sdata.2018.286.
37 A. Oehmichen, S. Jain, A. Gadotti, and Y. d Montjoye, “OPAL: High performance platform for large-scaleprivacy-preserving location data analytics,” in 2019 IEEE International Conference on Big Data (Big Data), Dec. 2019, pp. 1332–1342, doi: 10.1109/BigData47090.2019.9006389.
38 Les deux principaux piliers de la législation sur la protection des données personnelles dans l'Union Européenne sont le Règlement général sur la protection des données (RGDP), et la directive « Vie privée et communications électroniques » (e-privacy). Contrairement au RGPD qui est fondée sur la notion de gestion de risques, offrant ainsi des marges de
manoeuvre pour définir des solutions sur mesure et qui permet aussi des exceptions explicites pour le traitement des données personnelles, tels que l'intérêt public, la recherche scientifique ou les statistiques publiques, la directive e-privacy limite explicitement ces conditions, en interdisant aux opérateurs d'utiliser leurs données sans le consentement des clients.
39 Council of the European Union, Proposal for a Regulation of the European Parliament and of the Council concerning the respect for private life and the protection of personal data in electronic communications and repealing Directive 2002/58/EC (Regulation on Privacy and Electronic Communications). 2021.
Written by Stefania Rubrichi, Erwan Le Quentrec and Zbignew Smoreda
Extract of our white paper, Challenges and advancements in the era of data and artificial intelligence